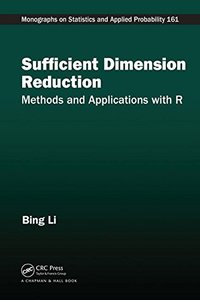
Sufficient Dimension Reduction: Methods and Applications with R (Chapman & Hall/CRC Monographs on Statistics & Applied Probability)
內容描述
Sufficient dimension reduction is a rapidly developing research field that has wide applications in regression diagnostics, data visualization, machine learning, genomics, image processing, pattern recognition, and medicine, because they are fields that produce large datasets with a large number of variables. Sufficient Dimension Reduction: Methods and Applications with R introduces the basic theories and the main methodologies, provides practical and easy-to-use algorithms and computer codes to implement these methodologies, and surveys the recent advances at the frontiers of this field.
Features
Provides comprehensive coverage of this emerging research field.
Synthesizes a wide variety of dimension reduction methods under a few unifying principles such as projection in Hilbert spaces, kernel mapping, and von Mises expansion.
Reflects most recent advances such as nonlinear sufficient dimension reduction, dimension folding for tensorial data, as well as sufficient dimension reduction for functional data.
Includes a set of computer codes written in R that are easily implemented by the readers.
Uses real data sets available online to illustrate the usage and power of the described methods.
Sufficient dimension reduction has undergone momentous development in recent years, partly due to the increased demands for techniques to process high-dimensional data, a hallmark of our age of Big Data. This book will serve as the perfect entry into the field for the beginning researchers or a handy reference for the advanced ones.
The author
Bing Li obtained his Ph.D. from the University of Chicago. He is currently a Professor of Statistics at the Pennsylvania State University. His research interests cover sufficient dimension reduction, statistical graphical models, functional data analysis, machine learning, estimating equations and quasilikelihood, and robust statistics. He is a fellow of the Institute of Mathematical Statistics and the American Statistical Association. He is an Associate Editor for The Annals of Statistics and the Journal of the American Statistical Association.